INTRODUCTION
Facilitators and barriers to tobacco product and nicotine addiction are highly varied1. While the actions of larger tobacco industry actors may garner more attention2, it may be valuable to assess the community-oriented effects of smaller actors that have direct relationships with tobacco-using communities. One type of smaller actor is the tobacco storefront, which can be defined as a retailer that physically exchanges tobacco products for money with the end user3,4. These tobacco storefronts can be subdivided into specialty retailers where tobacco products are the primary business line (e.g. vaping and smoke shops) and general retailers (e.g. grocery stores, convenience stores, chain retail outlets) where tobacco products are not the primary business line. Importantly, specialty tobacco retailers may represent an important geographical and demographic data point of community-level exposure and demand for tobacco product use.
Tobacco-specific storefronts have existed for several hundred years5. Yet, the last decade has seen the rapid popularization of a distinctly different subtype of tobacco-oriented retailer: the vape-specific storefront6. Generally, vaping products, also known as ‘electronic nicotine delivery systems’ (ENDS), are devices that use electricity to vaporize liquid, usually containing nicotine, which is then inhaled. While originally marketed as a cessation tool, vaping products have gained popularity, particularly among young adults7. Key drivers of uptake for young adults may not be cessation, but rather nicotine addiction, social pressure, and even competitive vaping8. In 2010, US national sales of ENDS were $11.6 million, increasing to $751 million in 20169. Increasing use of ENDS among youth and young adults has mirrored growing sales of ENDS9. In 2019, 23% of middle and high school students in the US were current tobacco users with 20% using e-cigarettes and 27.5% of high school students using e-cigarettes, the highest of any product10.
Reflective of the increase in the popularity of ENDS has been the proliferation of community-based vaping retail shops11. Importantly, vape shops concentrate on relational rather than solely transactional interactions with customers, focusing on developing rapport with customers and fostering a sense of community11-14. Vape shops are also important sources of information for current and potential ENDS users14,15. These shops may motivate their perception of ENDS as less harmful than conventional cigarettes16,17 and useful for cessation12,16,18, thereby impacting tobacco use attitudes and behaviors of customers. They also provide access to a wider array of products than non-specialty retailers11 and opportunity to sample e-liquids15.
Past work on the effects of the tobacco retail environment has shown that high tobacco retailer density is associated with youth smoking19-21. Furthermore, research has shown that retailer density is negatively associated with smoking abstinence and pro-cessation attitudes in poorer areas22. Other studies examining the retail environment of vape shops suggest that they are more concentrated near college and university campuses23 and where tobacco retail density is high24,25. Other community characteristics, however, have been shown to differ. In New Jersey, vape shops are located where few racial minorities live25. This is in contrast to vape shops in Orange County, CA, which are more common in areas with larger proportions of Hispanics24.
Less is known about the effects of retail density of vape shops on ENDS use, but these shops may play a significant role in shaping attitudes and norms around ENDS acceptability, initiation, and use26. Specifically, current ENDS use patterns suggest that age may be an important factor when attempting to measure the impact of community vaping retail outlets on tobacco and ENDS use behavior. This includes examining the increasing use of ENDS among youth and young adults. Hence, in this article, we use geographical data on tobacco-specific storefronts and vape-specific storefronts, along with geographically linked data on age groups, to better explore the relationships between retailer presence and separate age group tobacco use in California.
METHODS
Data collection
A list of names and addresses for currently-licensed tobacco retailers was obtained from the California Department of Tax and Fee Administration (CDFTA)27. This publicly available list details all licensed cigarette and tobacco product wholesalers, distributors and retailers licensed with the State of California, including retailers that sell vaping products. The list provides the following information: 1) license number; 2) business/taxpayer name; 3) doing business as (‘DBA’) name; and 4) address of licensee. For purposes of this study, we only examined the list of tobacco retailers current as of 16 May 2019. The public Application Programming Interface (API) for the search engine Microsoft Bing was used to obtain latitude and longitude coordinates for each business address included in the state-provided list of licensed tobacco retailers.
Scripts written in the programming language Python were then written to scrape the business directory service and crowd-sourced review forum Yelp in order to match retailer names and business addresses to Yelp registered business pages. This was done in order to further categorize our initial list of CA tobacco retailers into subcategories available in Yelp (e.g. tobacco shops, vape shops, grocery stores, convenience stores, etc.). Therefore, an interim dataset was assembled with each case being a licensed store having a Yelp page. This dataset contained variables which indicated whether the store was categorized in Yelp as a ‘tobacco shop’, ‘vape shop’, ‘grocery store’, etc. Our data collection process on Yelp allowed us to automatically match categories for 69% of retailers, with the remaining retailers manually categorized using existing Yelp categories. Yelp is a platform that has been used by other researchers to assess the discrepancies in vape-shop characteristics by demographic groups and also to assess differences in characteristics between vape shops that close or remain open after one year28,29. Data for the population of each age category and median age were obtained from the American Community Survey at the census tract level30. The final dataset assembled was structured in a manner whereby each case was a census tract, and variables included information about the number of stores in a given category (e.g. tobacco shop, vape shop, etc.) as well as age information for each census tract.
Statistical analysis
Our manual review of retailer Yelp pages (leveraging user-submitted images on Yelp and Google Maps) indicated that retailers categorized as ‘Tobacco Shops’ or ‘Vape Shops’ appeared to often be storefronts specialized in selling tobacco and/or vaping products, whereas other common categories of licensed tobacco retailers (e.g. ‘Gas Stations’, ‘Grocery Stores’, ‘Convenience Stores’) were non-specific vendors. Therefore, in this article, we refer to retailers categorized by Yelp as ‘tobacco stores’ as tobacco-specific, stores categorized by Yelp as ‘vape stores’ as vaping-specific, and all stores without these categorizations as ‘non-specific’. Though 22131 licensed tobacco retailers were provided by CDFTA, 1557 census tracts contained 1800 tobacco or vape shops, with only 200 containing more than one tobacco or vape shop. Therefore, geospatial analyses proceeded with three bivariate classifications of census tracts: 1) containing a tobacco-specific shop, 2) containing a vape-specific shop, and 3) containing either type of shop (Table 1).
Table 1
Number/proportion of shops that are vape-specific, tobacco-specific, both, and neither, based on Yelp categories, according to geography and highest ventile of each available age (years) category
Characteristics | Vape-specific shop | Tobacco-specific shop | Dual vape/tobacco shop | Non-specific tobacco retailer | |
---|---|---|---|---|---|
Sample size | Shops | 848 | 820 | 419 | 20320 |
Tracts with shop | 777 | 772 | 403 | 10837 | |
Tracts as percent of California | 3.4 | 3.3 | 1.7 | 46.7 | |
Tracts with 2+ stores | 65 | 44 | 16 | 5104 | |
Tracts in top ventile* (per capita) | Age under 5 (>10.9%) | 4.1 | 4.1 | 3.5 | 5.8 |
Age 5–9 (>10.1%) | 2.2 | 4.4 | 2.7 | 5.8 | |
Age 10–14 (>10.1%) | 3.0 | 2.7 | 3.5 | 4.8 | |
Age 15–19 (>10.6%) | 3.3 | 3.2 | 2.7 | 4.9 | |
Age 20–24 (>11.4%) | 8.8 | 8.2 | 6.7 | 5.5 | |
Age 25–34 (>24.9%) | 8.9 | 10.4 | 8.2 | 5.9 | |
Age 35–44 (>18.9%) | 3.5 | 7.0 | 4.0 | 4.6 | |
Age 45–54 (>19.8%) | 2.1 | 2.3 | 1.7 | 3.2 | |
Age 55–64 (>19.3%) | 2.1 | 2.1 | 2.2 | 3.9 | |
Age 65–74 (>13.0%) | 2.1 | 3.9 | 2.2 | 4.3 | |
Age 75–84 (>8.9%) | 4.0 | 5.4 | 3.2 | 4.6 | |
Age ≥84 (>5.0%) | 6.6 | 5.4 | 5.7 | 5.0 |
Age-related data and median age were available for 23194 census tracts in California. In addition, the populations for eleven age groups were available: age under 5 years, 5–9 years, 10–14 years, 15–19 years, 20–24 years, 25–34 years, 35–44 years, 45– 54 years, 55–64 years, 65–74 years, 75–84 years, and over 84 years. Total population was used to standardize across census tracts. Census tracts were then separated into ventiles of 1158 tracts for each age group per capita, allowing for continuousization of dichotomous characteristics as proportions of tracts containing each of the three dichotomous characteristics. For each age group, figures were produced to display the relationship between ventiles of age group proportion (see horizontal axes in Figure 1) and proportion of shops in each ventile of census tracts (see vertical axes in Figure 1), separately for vape shops and tobacco shops.
Figure 1
At the census track level, the relationship between ventiles of twelve separate age groups compared to percentage of tracts containing a vape or tobacco shop. Each ventile contains 1158 tracts
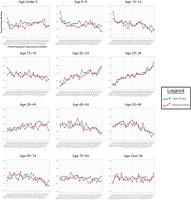
This study utilized a cross-sectional ecological design, whereby variables were reflective of retailer/ population characteristics in 2019, and analysis was conducted at the census tract level. In total, this study analyzed associations between retailer density and age groups at the census tract level using seventeen variables: 1) having a retailer which was tobacco-specific, 2) having a retailer which was vape-specific, 3) having a retailer which was both tobacco-specific and vape-specific, 4) having a retailer which was either tobacco-specific or vape-specific, 5) having a retailer which was neither tobacco-specific nor vape-specific, 6) proportion under 5 years old, 7) proportion 5–9 years, 8) proportion 10–14 years, 9) proportion 15–19 years, 10) proportion 20–24 years, 11) proportion 25–34 years, 12) proportion 35–44 years, 13) proportion 45–54 years, 14) proportion 55–64 years, 15) proportion 65–74 years, 16) proportion 75–84 years, and 17) proportion over 84 years. Least squares regression was used to test for significant linear relationships. A relatively low α=0.001 was used in assessing for statistical significance, as a high sample size reflected sufficiently high statistical power to detect very small effect sizes.
The high breadth of age representation among the dataset’s diverse set of census tracts suggested that a parabolic relationship (i.e., with older and younger age groups being significantly different from those in-between) may emerge between age and the presence of a tobacco or vape shop. Therefore, polynomial regression was used to test the relationship between ventiles of median age and proportion of tracts with vape/tobacco shops. Using the R package plotrix in R v3.6.0 (R Foundation for Statistical Computing: Vienna, Austria), graphing techniques were used to produce a dual-axis plot comparing the shape of the association of median age and presence of vape or tobacco shop with the association between median age and non-specific tobacco vendors. Tobacco-specific and vape-specific shops exhibited much lower frequency than non-specific shops. Therefore, since the magnitudes of these two typologies were very different, the utility of this visualization procedure enabled us to compare the shape of association for these two meaningful subsets of licensed tobacco retailers in California (Figure 2).
Figure 2
In black, the relationship between median age and shops which are categorized as either vapespecific or tobacco-specific, at the census track level. In red, the relationship between median age and shops which are neither categorized as vape-specific nor tobacco-specific
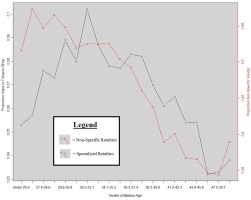
ArcGIS v10.7 (Esri: Redlands, CA) was used to produce maps that visualize the areas wherein age-related disparities appear most prominent. Point-coordinates of vape-specific storefronts and tobacco-specific storefronts are represented as distinct symbols atop a choropleth gradient map of age 25– 34 years per capita. Maps were produced for the four most populous cities in California along with their immediately surrounding areas, with all cities drawn on the same scale (Figure 3).
RESULTS
Visual assessment of the relationships between age category ventiles with the presence of both vape shops and tobacco shops conveyed a very strong, clear positive relationship for density of the age 25– 34 category and a somewhat strong, but also clear, positive relationship for density of the age 20–24 category (Figure 1).
Linear regression found statistically significant associations of age group ventiles with all three tract characteristics (i.e. containing a tobacco shop, containing a vape shop, and containing either) for density of four out of eleven age groups: 10–14 years, 20–24 years, 25–34 years, and 55–64 years. The characteristic of containing a vape shop also exhibited a significant linear association with age 5–9 years, and the characteristic of containing a tobacco shop additionally exhibited significant linear associations with age 15–19 years, age 35–44 years, and age 45–54 years.
Consistent with results in Figure 1, statistical procedures indicated significant negative associations with density of those aged 10–14 years, 15–19 years, 45–54 years, and 55–64 years, whereas the association between containing either a tobacco or vape shop was positive for age groups between the two extremes (e.g. younger than 19 or older than 45). The strength of the association was strongest for the positive relationship between ventiles of age 25–34 years and having either a tobacco or vape shop, with a range between 1.7% of tracts containing either a tobacco or vape shop for tracts at the lowest age ventile (under 5.5% population age 25–34 years) to 12.3% for tracts at the highest ventile (over 24.9% population age 25–34 years).
Polynomial regression found a significant inverse parabolic relationship between ventiles of median age and the proportion of tracts with tobacco or vape shops (p for linear term <0.001; p for quadratic term <0.001). Conversely, polynomial regression did not find a significant relationship between ventiles of median age and proportion of tracts with non-specific tobacco retailers (p for linear term = 0.375; p for quadratic term = 0.067). The shape of these distinct associations is visually overlaid in Figure 2.
Geospatial analyses exhibited clustering in three of the four most populous cities in California (Figure 3). In Los Angeles, several clusters of many vape/tobacco shop concentrations seemed to exist, with perhaps the most distinct around Koreatown. In San Diego, a cluster appeared to exist along a portion of El Cajon Blvd about 2–3 miles south of San Diego State University. In San Jose, clear clustering was not apparent, although a relative dearth of vape and tobacco shops appeared to exist in neighboring Milpitas and Sunnyvale. In San Francisco, one notable cluster emerged across the agglomeration of businesses spanning Tenderloin and the Financial District.
DISCUSSION
Our study using validated addresses of licensed tobacco and vaping shop retailers showed that areas with high numbers of specialized tobacco/vaping retailers had high numbers of young adults, and this relationship was not observed for other age groups. These findings may indicate that tobacco and vaping specialty retailers are focusing on this young adult age demographic (20–34 years) for product marketing and access, and community-based engagement.
According to a 2014 report from the US Surgeon General, the average age of smoking initiation was 15.3 years31. Therefore, this study may elucidate a timeline for increasing smoking intensity whereby occasional use in youth and adolescents leads to more sustained tobacco behavior in later more advanced young adult years, with vendors responding to this demand by operating their businesses in areas with a higher distribution of this age demographic. It likely also reflects the enforcement of the minimum age to purchase tobacco products in the state, which was increased from 18 to 21 years in 2016. Conversely, the relatively muted relationship between density of age 15–19 years with the presence of a tobacco or vape shop may relate to other factors, such as inaccessibility caused by teenagers living with their parents or college bans of tobacco and vape products.
For example, a large number of college campuses have banned consumption of tobacco and vape products, including the 9 campuses of the University of California and the 23 campuses of the California State University32. It is possible that the effect of these policies may serve as a minor contribution to explaining the null association observed between density of the 15–19 age group and presence of a tobacco or vape shop, and perhaps may also help to elucidate the relatively muted relationship with density of the 20–24 age group when compared to the somewhat clearer relationship observed among the 25–34 age. Further research should be conducted to determine whether an expansion of targeted tobacco control policies (e.g. restrictions on product marketing) for geographies with relatively dense concentrations of the age 25–34 group would benefit tobacco control efforts in California.
Little difference was observed between the relationship of age variables between tobacco-specific shop presence and vape-specific shop presence. This may indicate that age-dependent differences stratified for different types of tobacco products may be difficult to observe in ecologically focused studies that use geographical location as the primary predicting variable. It may also indicate that age demographics may be skewed by other tobacco use factors, such as poly tobacco use or transition between combustibles and ENDS use in this population33,34. Future research should focus on identifying geographically bound age-related associations for tobacco use stratified for specific tobacco products, while also assessing other sociological variables (e.g. sex, socioeconomic, race) that may influence initiation, use and transition.
Limitations
This study should be considered primarily as hypothesis generating, as its ecological methodology does not permit attribution of findings to individuals. Specifically, the significant positive relationship between the concentration of those aged 25–34 years and the proportion of tobacco-specific or vape-specific shops in census tracts, does not necessarily indicate that individuals in the age 25–34 group are more likely to use the products most associated with these relatively specialized shops. Moreover, though these shops are known to carry a broader array of tobacco and/or vape products, young adults shopping at these stores may be purchasing the same products in non-specific retailers that carry the same tobacco or vape products. In addition, as this study leveraged categories listed on Yelp, it relies on a retail owner’s self-categorization of ‘Vape Shop’ or ‘Tobacco Shop’ labels, which could be subject to inconsistencies. However, the potential effect of any misclassification was minimized by our manual review of user-submitted images for these shops. Finally, this study attributes licensed tobacco retailers as epidemiologically meaningful with respect to potential consumer uptake of ENDS compared to other tobacco products. However, more rigorous research needs to be conducted to compare whether users most commonly obtain specific types of vape or tobacco products from these retailers, online stores, or other outlets.
CONCLUSIONS
This study adds hypothesis-generating information about disparities in spatial risk for tobacco/vaping use between different age groups. Use of vaping products is unevenly distributed among demographic groups, and this disparity may be perpetuated by an uneven distribution in storefronts that specialize in the sale of these products. Results of this study show that tobacco and vaping storefronts were much more commonly found in areas with higher concentrations of those aged 20–34 years, but this association was not found for younger or older age categories. These findings emphasize the need for anti-tobacco efforts to target groups that are at especially heightened risk for e-cigarette uptake and use, and these findings underscore the particular importance of generational differences in tobacco-related behavior.